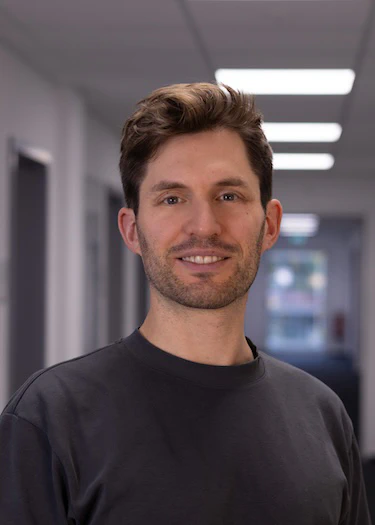
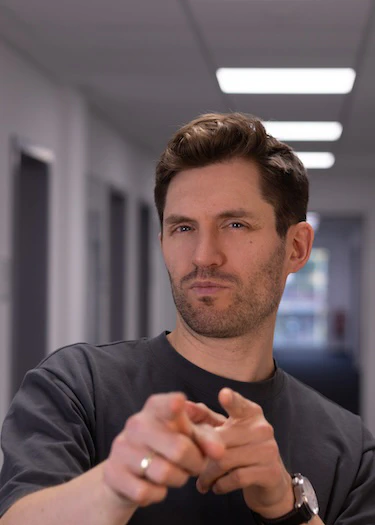
Dr. Nils Winter
PI Junior Research Group Precision Psychiatry
Contact Nils Winter
Google Scholar
My research lies at the intersection of neuroscience, machine learning, and clinical psychiatry. With a background in cognitive neuroscience and clinical psychology, I am particularly interested in how computational methods can help us better understand, predict, and eventually intervene in mental disorders. I approach psychiatric illnesses as complex, dynamical systems and combine data-driven modeling with theoretical perspectives to uncover the mechanisms underlying individual symptom trajectories.
As the head of the Precision Psychiatry research group, my work is organized around three main areas. First, I develop and apply machine learning models for individualized clinical prediction, aiming to support decision-making in psychiatry—for example, by predicting relapse risk or treatment response. Second, I use neuroimaging data, particularly structural and resting-state MRI, to identify biomarkers and network-level alterations associated with psychiatric conditions such as major depressive disorder. A core focus here lies in connectome-based modeling and the integration of biological information with behavioral phenotypes. Third, I explore formal models of psychopathology, drawing from computational neuroscience to better characterize psychiatric disorders as dynamical systems.
Across all of these areas, I advocate for open, transparent science and contribute to the development of open-source research software, including PHOTONAI, a machine learning framework tailored to the needs of biomedical researchers.
I am also an active member of the Otto Creutzfeldt Center for Cognitive and Behavioral Neuroscience (OCC ), where I engage in interdisciplinary exchange on topics at the intersection of brain, behavior, and computation.
Projects
Biomarkers for Major Depressive Disorder
Considering that biological psychiatry is built on the premise that mental disorders have a neural basis, it is essential for the field to derive biomarkers of MDD informative on the level of the individual patients.
Read MoreA Python Toolbox for Connectome-Based Predictive Modeling
Confound-Corrected Connectome-Based Predictive Modelling is a Python package for performing connectome-based predictive modeling (CPM).
Read MoreControlling Confounds in Biomarker-Based Clinical Prediction Models
In recent years, brain-based clinical prediction models have shown great promise in identifying biomarkers for psychiatric and neurological disorders.
Read More